The Strategic Formation Game in Football
The modern sport of European football has its root
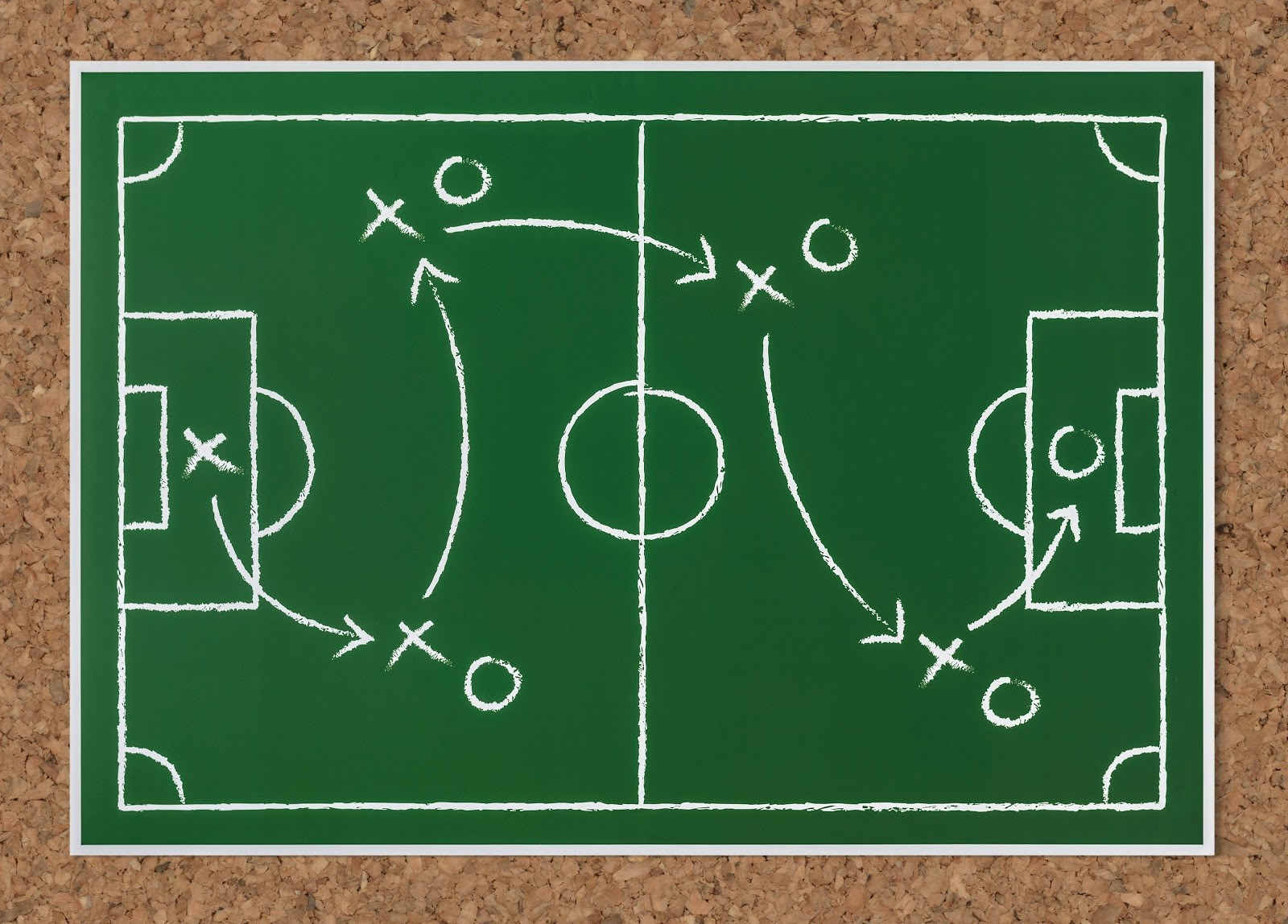
The modern sport of European football has its roots in medieval Britain. The rules varied by place. They had no set formations or positions. Players could even handle the ball. The game involved two teams. They tried to force a ball into the other team's goal. Football was a violent game and was even reportedly outlawed at times. It didn't look like the game played today. It was head-down charging. Teammates backed up the player with the ball. The first set of standardized rules, the "Cambridge Rules," was published in 1848. For more historical insights like these, check out Blog Vave.
Data Set: Team Formation and Team Strength
We use Hugo Mathien's European Soccer Database on Kaggle. It includes over 25,000 matches from top leagues in 11 European countries (2008-2016). This dataset combines real match data with player attributes from FIFA.
For our study, we focus on goals. We analyze team formations by looking at where starting players played. Since the dataset doesn't specify formations, we derived them from players' starting positions. We removed matches without final scores or player positions, leaving 21,360 games.
Each FIFA player record has 34 attributes. We sum starting players' Overall Ratings (0 to 100) to determine team strength. This rating, calculated by EA Sports, is based on relevant skills.
Using these ratings, we compare teams in each match. Our database includes goals scored, formations used, and team strengths.
We examine home-field advantage and team strength. These features are linked to goal difference. They are key for the game-theoretical models in Section 4.
Home Field Advantage
Football acknowledges a home-team advantage, and our data supports this. The differences in final scores follow a Gaussian distribution. They show up in the data. The mean is above 0. The home team wins 45.9% of matches; the away team wins 29.8%, and 24.3% ends in a draw.
The goal difference distribution is not affected by team strength. All teams play each other once at home and once away. Figure 1b shows this symmetry. It displays the strength ratio. The home team's strength is divided by the away team's strength.
The Strategy Set and Game Theory Application in Formation Selection
Game theory studies strategy and optimal decision-making among competing players. Players maximize their payoffs by considering their identities, preferences, and available strategies. Many fields, including psychology, biology, politics, and business, use it.
A player's strategy set includes all pure strategies they can choose from. A mixed strategy assigns probabilities to each pure strategy, allowing for random selection. A Nash equilibrium is a solution. No player can benefit by changing their strategy if others keep theirs unchanged. Nash proved that every game has at least one Nash equilibrium. This is true for games with finite players and pure strategies. If we allow mixed strategies, it
In this study, we model football formation selection using game theory. We focus on the most common 5x5 formation pairs from a dataset of 24 formations. This reduces the combinations from 576 to 25. It causes minimal data loss, only 7,000 out of 21,000 matches.
The selected formations are:
- 4-4-2 (most common)
- 4-2-3-1 (second most common)
- 4-3-3 (recently very popular)
We chose these formations because each pair appears in at least 20 matches.
Modeling Strength-Agnostic and Strength-Aware Formation Games in Football
We assume that teams can choose from 5 formations. They will analyze 25 strategies based on historical data. We look at two scenarios:
- Strength-Agnostic Formation Game: Removes team strength bias to model similar-quality teams.
- Strength-Aware Formation Game: Payoffs are partly determined by team strength relations.
Strength-Agnostic Formation Game
To find the best formation, we add final scores for all selected pairs. Then, we create a payoff function that removes the strength effect. Based on strength, teams were divided into five large groups.
We use a normalized scoring system: 1 point for the winner, 0 for the loser, and 0.33 points each for a draw. Our payoff function adjusts points based on the team strength ratio. Using a linear regression model, we predict the expected goal difference. If a prediction error occurs, reward the winning team and penalize the losing team.
Classification of Matches in the Strength-Aware Formation Game
We divide matches into three groups based on the team strength ratio.
1. Weak Home Team vs. Strong Away Team (W-HT vs. S-AT)
Strength Ratio (SR) less than 0.97 (SR < 0.97)
2. Strong Teams (E-HT vs. E-AT)
Strength Ratio between 0.97 and 1.03 (0.97 ≤ SR ≤ 1.03)
3. Strong Home Team vs. Weak Away Team (S-HT vs. W-AT)
Strength Ratio greater than 1.03 (SR > 1.03)
Analysis of Strength-Aware Game Theory in Football Formations
In the strength-aware game, we use normalized scoring: 1 point to the winner, 0 to the loser, and 0.33 points each for a draw. We create payoff matrices for three groups of matches.
1. Weak Home Team (W-HT) vs. Strong Away Team (S-AT):
- Mixed Nash equilibrium: W-HT plays 4-3-3 (43%) and 4-4-2 (57%); S-AT plays 4-3-3 (65%) and 4-4-2 (34%).
- Stronger away teams play more, but home-field advantage still influences formation choices.
2. Equally Strong Teams (E-HT vs. E-AT):
- Mixed Nash equilibrium: E-HT plays 4-2-3-1 (65%) and 4-5-1 (35%); E-AT plays 4-3-3 (40%) and 4-4-1-1 (60%).
- E-HT prefers the favored 4-2-3-1, while E-AT opts for a defensive 4-4-1-1 due to home-field advantage.
3. Strong Home Team (S-HT) vs. Weak Away Team (W-AT):
- Mixed Nash equilibrium: S-HT plays 4-3-3 (64%) and 4-4-2 (36%); W-AT plays 4-4-2 (98%) and 4-5-1 (2%).
- S-HT is often offensive, while W-AT tends to play.
Our game theory results agree with modern football trends. Flexible formations, like 4-3-3 and 4-2-3-1, offer the best payoff. Starting formations do not show the whole match. Yet, they give critical insights into game planning.
Final Thoughts
Selecting the right team formation is crucial in football. In this paper, we use machine learning and game theory. We use them to investigate the selection of strategic formations. We analyze over 25,000 matches, focusing on two scenarios:
- Strength-Agnostic Formation Game: Removes team strength bias. We find that 4-3-3 against 4-3-3 is a pure Nash equilibrium.
- Strength-Aware Formation Game: It considers team strength relations. Mixed Nash equilibria are presented and analyzed.
Our models align with modern football trends. They show that flexible formations like 4-3-3 and 4-2-3-1, plus the classic 4-4-2, yield the best payoffs.