How to use Advanced Analytics to boost your football betting strategy
In the ever changing world of football betting adv
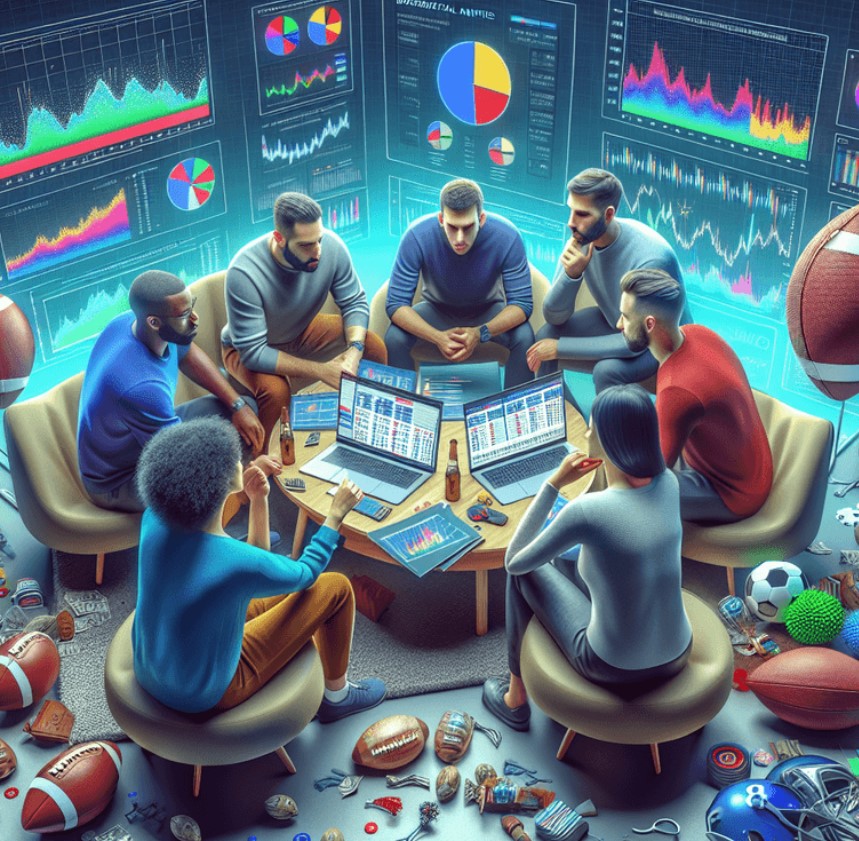
Introduction
In the ever changing world of football betting advanced analytics is the new game changer. Using data driven methods allows you to make better decisions and gain an edge.
Traditional betting methods are based on gut feel or basic stats. Advanced analytics goes deeper into the numbers and finds patterns and insights that were previously hidden. By using these you can improve your betting strategy and win more.
GemBet and other betting platforms offer many ways to incorporate advanced analytics into your approach. The main point is clear: using data driven methods can turn your football betting from guesswork to fact.
Advanced Analytics in Football Betting
What is Advanced Analytics?
Advanced analytics uses complex methods and algorithms to analyze large amounts of data, to provide practical insights. In football betting this means using data analytics to look at past performances, player stats and other key factors to predict future results.
How does it affect modern betting?
- Data Driven Decision Making: Unlike traditional betting which is based on gut feel or basic stats, advanced analytics uses full data models. So you can make decisions based on facts not feelings.
- Better Predictive Accuracy: By using machine learning and statistical modeling advanced analytics can find patterns and trends that are not visible to the naked eye. So predictions are more accurate.
How is it different from traditional betting methods?
- Data Over Intuition: Traditional methods are based on gut feel or anecdotal evidence. Advanced analytics is based on data interpretation.
- Complex Algorithms vs Basic Stats: Traditional betting uses simple metrics like win loss records, advanced analytics uses complex algorithms like regression analysis and Monte Carlo simulations.
- Continuous Improvement: Advanced analytics frameworks are dynamic and get better with more data. Traditional methods are not.
By reading this you can see how advanced data analytics gives you an edge in football betting.
Statistical Analysis Techniques to learn
Statistical analysis is key to a solid football betting strategy. By learning these methods you can analyze large amounts of data and make predictions.
1. Regression Analysis
Regression analysis is a powerful tool to understand relationships between variables. In football betting it helps to identify factors that affect match outcomes. For example:
- Linear Regression: This looks at the relationship between two variables, like team performance and match result.
- Logistic Regression: Used for binary outcomes like win/loss, logistic regression predicts the probability of a specific outcome based on multiple predictors.
2. Monte Carlo Simulations
Monte Carlo simulations involve running many simulations to model the probability of different outcomes. This is useful in football betting as it can incorporate randomness and variability. Key benefits:
- Risk Assessment: By modeling multiple scenarios you can assess risk and reward.
- Outcome Probability: Helps to estimate the probability of different match results based on historical data.
3. Predictive Modeling
Predictive modeling is key to forecasting match outcomes. It involves using historical data to build models that predict future events. Within predictive modeling there are several techniques that are important for football betting:
- Machine Learning Algorithms: Methods like decision trees, random forests and neural networks can find patterns in data.
- Feature Engineering: Selecting the right features (e.g. player stats) will improve the model.
Predictive models allow you to forecast match results with more accuracy. For example by looking at Expected Goals (xG) and Expected Assists (xA) a model can predict how likely a team is to score or assist based on past performance.
Now you have the statistical analysis techniques to make data driven decisions in football betting.
Data Driven Metrics
Key Player Stats
To make betting decisions you need to track these key player stats:
- Expected Goals (xG): This metric estimates the probability of a shot becoming a goal based on factors like shot angle, distance from goal and type of assist received. By looking at xG you can measure a player’s scoring ability beyond just goal count.
- Expected Assists (xA): Similar to xG this metric looks at the quality of the pass that leads to a shooting opportunity. It allows you to identify playmakers who contribute most to their team’s attack.
- Pass Completion Rate: This stat gives you insight into a player’s ability to maintain possession and create plays. High pass completion rates mean strong midfielders who can control the tempo of the game.
- Dribbles Completed: Tracking successful dribbles helps you assess a player’s ability to beat defenders and create scoring opportunities. Players with high dribble completion rates are usually the ones who break down the opposition’s defense.
Team Factors
Team dynamics are just as important for match outcomes:
- Team Chemistry: The cohesion between players can have a big impact on match results. Data on player interactions (e.g. passes between certain players or defensive partnerships) gives you insight into team chemistry.
- Formation Analysis: Different formations can affect a team’s performance based on their strengths and weaknesses. Tracking how teams perform in different formations helps you predict against different opponents.
- Injury Reports: Injuries disrupt team dynamics and can change match predictions. Make sure you have up to date injury data for more accurate betting strategies.
- Home vs Away Form: Teams perform differently at home and away. Analyse historical data on home and away form to understand these differences.
Using analytics to track player stats and team dynamics gives you all the data you need to make better betting decisions. Now use these insights to predict match outcomes.
Building a Predictive Model for Betting
Step by Step Guide to Building a Model
Building a model for match outcome prediction involves several steps.
1. Data Gathering
Gather historical data like team stats, player stats and match outcomes. Make sure the data is clean and relevant, remove any inconsistencies or outliers.
2. Feature Engineering
Determine which variables (features) have the most impact on the outcome. Common features are:
- Team form: recent stats
- Player injuries and suspensions: availability of key players
- Home and away stats: performance variation based on venue
- Head-to-head: historical results between the two teams
3. Algorithm Selection
Choose an algorithm based on your data type and prediction goal. Popular algorithms for football betting are:
- Logistic Regression: Good for binary outcomes like win/loss
- Random Forest: Handles big datasets and complex interactions well
- Gradient Boosting Machines (GBM): Great for iterative refinement of accuracy
Train your model on historical data then test it on a separate dataset to ensure it generalises well to new, unseen data.
Limitations and Caveats
While models can help your betting strategy, they have limitations.
Overfitting
Overfitting is a common problem where your model performs well on training data but poorly on new data. Fix this by:
- Using cross-validation
- Simplifying your model by removing less important features
- Regularising your model to penalise complex solutions
Market Efficiency
Market efficiency is also a problem. Betting markets are influenced by many variables that models can’t account for. Continuous monitoring and updating of your model is key to account for new trends and changes in team dynamics.
Building a robust model requires attention to detail in data gathering, feature engineering and algorithm selection. While overfitting and market efficiency are limitations, knowing these will help you improve your approach.
Beyond the Numbers: External Factors in Betting
While analytics provides a solid framework for decision making, external factors can have a big impact on match outcomes and shouldn’t be ignored. These factors often add layers of complexity beyond what data can predict.
Weather
Weather can have a big impact on football matches. Rain can make the pitch slippery, affecting ball control and player stability. Strong winds can change the trajectory of the ball, making aerials and long passes complicated. Bettors should consider:
- Rain: More defensive errors and fewer goals.
- Wind: Passing accuracy disrupted and unexpected goals.
- Temperature: Extreme heat or cold can affect player fitness and performance.
Player Injuries
Injuries to key players can change the team dynamics. Understanding the impact of these injuries means:
- Key Player Absences: Loss of top scorers or defenders can weaken the team.
- Team Morale: Injuries can demoralise teams and lead to poor performances.
- Replacement Quality: Assessing the quality of the substitute players is key.
Other External Factors
Other factors also apply:
- Home/Away Advantage: Home teams tend to perform better due to familiarity and fan support.
- Managerial Changes: New manager can bring unpredictable changes to team performance.
- Travel Fatigue: Long distance travel for away games can affect player energy levels.
Including these external factors in your betting strategy gives you a more complete picture, and complements the insights from data driven analytics.
Finding Value Bets Through Data
Value bets are where the bookies odds are higher than the actual probability of the event occurring, so you can exploit the market inefficiencies. Advanced analytics can help you find these opportunities.
Practical Tips to Find Value Bets
1. Collect and Analyse Historical Data
- Get data on past performances, head-to-head stats, team form and player metrics.
- Use Python or R to clean and analyse this data.
2. Implied Probabilities
- Convert bookie odds into implied probabilities using the formula:
- [ \text{Implied Probability} = \frac{1}{\text{Odds} } ]
- Compare these to your own probabilities from statistical models.
3. Use Predictive Models
- Run predictive models such as regression analysis or machine learning algorithms to estimate the probability of different outcomes.
- For example, run a logistic regression to predict the probability of a team winning based on xG (expected goals), recent form and player conditions.
4. Market Movement
- Track the odds over time and look for patterns that indicate market inefficiencies.
- Betfair’s historical data is very useful for this.
5. Adjust for External Factors
- Include external factors such as weather, injuries and managerial changes in your models.
- For example, if a star player is injured, adjust your probabilities accordingly.
Real World Examples
- Underdog Wins: Analyse past data and you might find that certain underdog teams perform well in certain situations (e.g. home against top teams). If bookies undervalue these scenarios, you have a value bet.
- In-Play Betting: During live matches use real-time data to find value bets. For example, if a team is dominating possession and creating chances but haven’t scored yet, their winning odds might be value if they are still underdogs.
By using these methods you can find value bets and make informed decisions. This will increase your chances of winning and help you manage risk.
Using Analytics for Long Term Football Betting Success
Using analytics in your football betting strategy is key to making consistent profits. By using data driven methods you can make better decisions and reduce the impact of bias and guesswork.
Points to remember:
- Better Decision Making: Analytics will help you see patterns and trends that traditional methods will miss.
- Long Term Profits: Using analytics regularly will lead to long term success not luck or gut feeling.
FAQs
What is advanced analytics in football betting?
Advanced analytics is the use of data driven methods and statistical techniques to inform decision making in football betting. It’s different to traditional methods by using quantitative analysis rather than intuition or anecdotal evidence.
What statistical analysis techniques are used in football betting?
Key statistical analysis techniques for football betting are regression analysis, Monte Carlo simulations and predictive modeling. These help bettors forecast match outcomes and understand trends by looking at historical data.
How do player performance metrics affect betting decisions?
Player performance metrics such as xG and xA give insight into individual player contribution. By tracking these metrics bettors can make decisions that reflect a player’s true impact on match outcome.
What to consider when building a predictive model for football betting?
When building a model consider data selection, feature engineering and potential limitations like overfitting and market efficiency. A good model will improve prediction accuracy.
Why are external factors important in football betting?
External factors like weather, player injuries and matchday dynamics can have a big impact on match outcomes. Including these in your betting strategy will give you a more complete view of the outcome.
How do I find value with advanced analytics?
To find value bettors should look at market inefficiencies by comparing their predictions to the bookmaker’s odds. Practical tips are to use statistical models to calculate probabilities and look for the gap between expected outcome and available odds.